There’s nothing new about data reduction in storage systems, right? WRONG! While it’s easy to treat various data reduction techniques as checkboxes on a long list of features, the old adage of “The devil is in the details” could never be truer.
Here at VAST Data we’re hard at work on a never-ending mission to innovate and develop new and improved techniques that enable our customers to fit more logical data into less physical space than ever before. In my role as Principal Technical Marketing Engineer at VAST, I have the opportunity and lab gear to test and implement many of these techniques, some of which can be real eye-openers for veteran IT folks who have already “seen it all” … until now.
“All Data Reduction is Not Created Equal,” a new white paper spearheaded by my colleague Howard Marks, captures these key VAST data reduction techniques and innovations. Howard does an excellent job breaking down complex data reduction concepts into simpler terms that help us wrap our heads around what they are, how they work, and why they matter. If you’ve not yet read that paper, I strongly encourage you to do so as it’s educational and insightful for both storage novices and seasoned storage vets like Howard.
Data reduction techniques not only help drive down the effective price per terabyte and improve overall economics, there are additional benefits not often talked about during the purchasing process. For starters, let's divide up all our data and use cases for those into two camps:
Huge datasets expected to be unique
Huge data sets where we expected to see lots copies of the similar data.
In the first camp, the huge unique datasets, the goal is to reduce that unique data as much as possible. Techniques like compression work well here, while more advanced forms such as Data-Aware Compression reduce it even further. While data-aware compression may not be new for files, applying it automatically and in real-time at the storage layer is a ground-breaking data reduction innovation from VAST.
But even in this camp where your data set contains lots of unique files without overlaps of common data, conventional wisdom would argue: “Deduplication would be an unnecessary overhead and there is no opportunity to reduce data within large sets of unique data.” They may argue that compression is all that is required and anything beyond that is a waste of compute.
I disagree and believe that compression alone isn’t enough.
Why? If for no other reason, let me go with “people are messy.” Or “people are paranoid.” Or maybe “people are messy AND paranoid.” And by this I mean that users tend to access data from one share, and then keep a copy in another. DBAs (database administrators) in particular are often guilty of making their own copies when they don’t trust systems are being backed up adequately by administrators. Without data deduplication techniques, organizations pay a penalty for this behavior.
What about the messy people? Maybe “messy” isn’t the best description. Take an individual who found an interesting set of data, but it doesn’t quite work well in their analytical tools of choice. So they make a copy, transform it in some way or another, and then perform some analysis. And then that analysis is output to yet a different format. Within every step, they have created new data that is very similar to the original data set. While there is opportunity for like data to be matched to like data, legacy deduplication techniques aren’t up for that task. Enter VAST and its innovative techniques to once again help organizations avoid this penalty.
And that other camp… the one expecting a lot of duplicates? Let’s just lump that into a category commonly referred to as “Data Protection.” VAST clusters make for an AWESOME data protection target for your favorite backup software. You might be thinking “Duh! Of course it’s awesome! I’d love to have an all-flash storage repo for my backups. I’d also love to drive myself from point A to B in a Ferrari… but my budget dictates otherwise.” Are you sure? Have you done the math? I’ll agree with you on the Ferrari... but let’s dig in a bit deeper on how VAST helps the data protection use cases. You may be pleasantly surprised to find out this Ferrari magically has the trunk capacity of a freight train with 1000 mpg fuel efficiency. Have you seen the price of gas lately?!?! Time to take another look… 😉
Onwards!
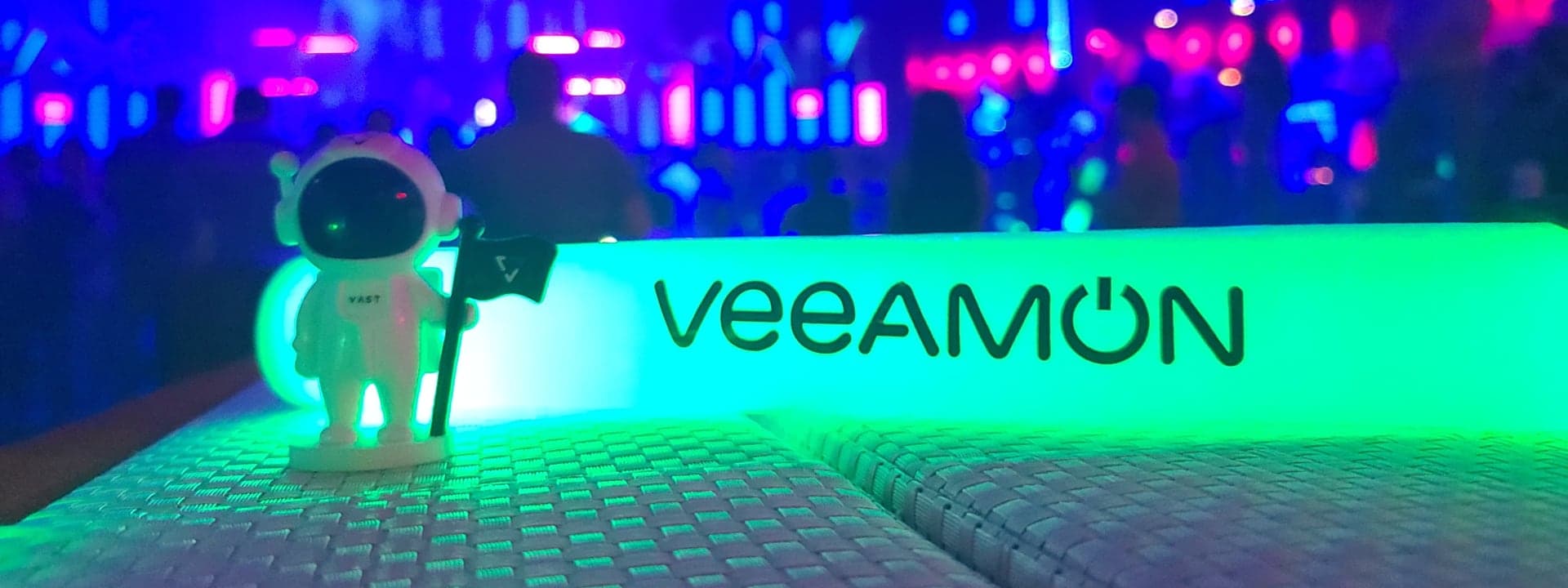
A few months ago, we sponsored VeeamON and I was lucky enough to attend. It was great to be back at an in-person trade show! For months leading up to the event, I was really busy in the lab, testing out a number of backup and restore scenarios to ensure I’d have answers and good advice to provide attendees and partners that I’d surely be conversing with at the show. I was also very busy testing and validating a new data reduction technique that was going into the beta of VAST v4.3 launching around the same time as the show. That new technique is what we now refer to as “Adaptive Chunking.” It was incredible how much more savings I got with adaptive chunking over existing VAST data reduction methods (which were already quite remarkable!). Refer back to our white paper for an in-depth look at adaptive chunking.
Little did I know, things were about to get even more exciting AFTER the show. On the heels of VeeamON were a lot of follow-up conversations and deployments. Not only did adaptive chunking have huge potential to find better matches with its byte-granular hashing technique, but these savings were being realized in the field. We immediately saw an uptick in DRR (data reduction rates) across systems that had upgraded to 4.3.
In working with customers to deploy their backup solutions, one of the things we found was a performance uptick from increasing the blocksize. With traditional data deduplication techniques, larger blocks result in the backup software getting reduced efficiency, but not with VAST… anything that the backup software may have reduced was in fact being further reduced by the VAST cluster.
But that’s not all…
I also stumbled upon a capacity penalty for changing the deduplication blocksize from within the backup software. After changing the blocksize, software-dedupe is only effective with a new base (ie. full backup) since all of the hashes are different. Now this presents a double-penalty: MORE data needs to be written (100% of the base backup size), AND if your storage isn’t performant, there’s a big time hit waiting for that full backup to complete. Again, VAST to the rescue to nullify that penalty.
At this point, you may be saying to yourself: “Ya… we get it… VAST reduces data better than anyone.” But I haven’t even gotten into performance! Not only are we reducing data in real-time, but we’re serving it up FAST! How fast? About 8x our ingest speeds! So not only do we make a great target to back up to, but when you really need that data back, we’re serving it up as fast as your data movers can retrieve it and write it to the restore target(s).
Oh, what’s that? Your restore target doesn’t have enough capacity to restore everything? Why not write it back into the same VAST cluster the backup files live in? After all, we’re on VMware’s vSphere 7 HCL. That’s right - we’re a fully functional datastore, not just a great place to dump a lot of data. Remember all that stuff I was raving about with regards to amazing space savings? It’s ready to pay dividends yet again because restoring to the same cluster requires only a fraction of the physical capacity it would otherwise require to write the logical full copies elsewhere. These live datastores also make a great home for all the critical backup software infrastructure VMs to live, complete with their own protection leveraging VAST’s snapshot & replication capabilities.
At risk of writing too long a novel that no one will get to the end of, I think I’ll end it here for now. Every blog done right always ends with a call to action, so here it is: Contact us to learn more ways we can help you manage and protect your data. And if you’re skeptical about us having the BEST data reduction in the industry, reach out to your local VAST rep to get a copy of our probe to analyze just how much we can reduce your data if it had the good fortune to live on a VAST cluster. Until next time…
—Rob